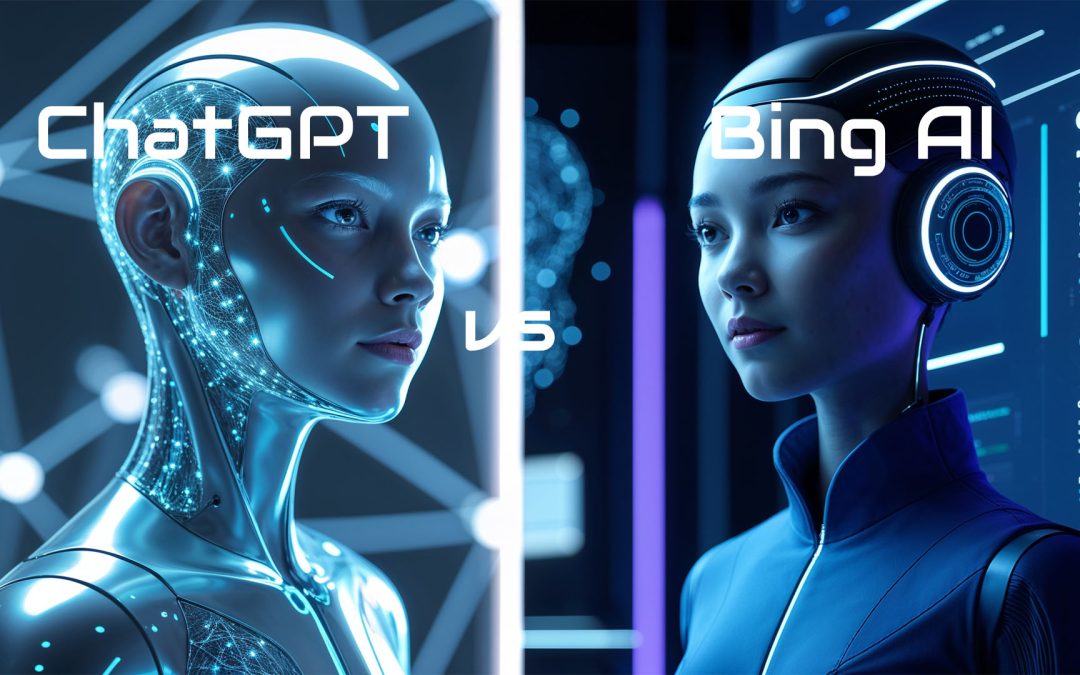
ChatGPT vs Bing AI: Ultimate Showdown of Smart Assistants in 2025?
ChatGPT vs Bing AI – Introduction
Artificial Intelligence is no longer a concept of the future—it’s already here.
Among the frontrunners shaping our daily interactions with AI are ChatGPT (by OpenAI) and Bing AI (powered by Microsoft’s integration of GPT-4).
But how do they compare in real-world usage, power, ethics, accessibility, and performance?
This comparison examines both assistants’ core capabilities, social integration, tools, speed, and limitations, helping you decide which AI better meets your needs.
1. Origins and Technology
ChatGPT:
- Developed by OpenAI.
- Available in multiple versions (GPT-3.5 for free users, GPT-4 for Plus users).
- Chat-centric interface, versatile in reasoning, writing, coding, and storytelling.
Bing AI:
- Built by Microsoft, based on OpenAI’s GPT-4 but with extra layers.
- Fully integrated into Bing search and Microsoft Edge.
- Combines chat with live web access and visual answers (via DALL·E).
2. Access and Pricing
Feature | ChatGPT | Bing AI |
---|---|---|
Access | Web + mobile (OpenAI) | Web via Bing/Edge browser |
Free Tier | Yes (GPT-3.5) | Yes (GPT-4 base + web) |
Premium | $20/month (GPT-4) | Free (but limited by conversation) |
3. Web Access and Freshness
- ChatGPT (as of GPT-4o): browsing is optional and can be turned on/off.
- Bing AI is always connected to the web, offering real-time search summaries.
Result:
For up-to-the-minute facts or current events, Bing AI may edge ahead. But ChatGPT’s controlled browsing is more refined for research.
4. Interface and Usability
ChatGPT:
- Streamlined UI, suited for deep conversations.
- Code interpreter, memory features, and plugin/store integration.
Bing AI:
- Feels more like a traditional search engine but with added conversational capability.
- Limited by chat turn quotas and sometimes overly formal responses.
5. Social Integration & Ecosystem
ChatGPT:
- Used widely across developer communities, educators, and creatives.
- Integrates into Slack, Zapier, and numerous productivity apps.
- Lacks built-in social or search engine presence.
Bing AI:
- Deeply embedded in Microsoft’s ecosystem (Edge, Windows 11 Copilot).
- Can answer directly inside search results, ideal for web-native users.
- Shares visual responses in social contexts (image generation with DALL·E).
6. Speed and Responsiveness
- ChatGPT-4o (2024 release) is extremely fast, even in complex reasoning.
- Bing AI often pauses or “thinks” before giving a reply.
7. Creativity and Tone
- ChatGPT is more fluent in long-form writing, natural storytelling, coding, and poetic tone.
- Bing AI feels more “search assistant-like”, with structure but less warmth.
8. Limitations and Risks
Limitation | ChatGPT | Bing AI |
---|---|---|
Hallucination Risk | Present but mitigated by browsing | Still present, even with real-time |
Source Citation | Manual | Automatically embedded |
Censorship | Moderate filters | Tighter content control by Microsoft |
9. User Trust & Feedback
- ChatGPT is perceived as more independent, with growing trust among coders, students, and writers.
- Bing AI is seen as a search enhancer, praised by casual users but less trusted by professionals.
❓ 10 FAQs – ChatGPT vs Bing AI
1. What is the main difference between ChatGPT and Bing AI?
A.: ChatGPT is focused on deep conversations and creativity. Bing AI integrates search capabilities and web-based answers.
2. Does ChatGPT have internet access?
A.: Yes, with GPT-4o browsing enabled. Users can toggle web access when needed.
3. Is Bing AI better for real-time information?
A.: Yes. Bing AI continuously pulls in fresh data from the web.
4. Can ChatGPT be used for programming?
A.: Absolutely. It supports code generation, debugging, and interactive Python execution.
5. Which one is better for students?
A.: ChatGPT is better for essays and explanations; Bing AI is good for quick lookups.
6. Are both AIs based on GPT-4?
A.: Yes, but Bing AI uses a version of GPT-4 optimized by Microsoft.
7. Can I use them on mobile?
A.: Yes. Both ChatGPT and Bing AI have mobile apps or browser access.
8. Do they remember previous chats?
A.: ChatGPT Plus with memory can remember context; Bing AI has short memory per session.
9. Do they generate images?
A.: Bing AI integrates DALL·E. ChatGPT can use DALL·E too via OpenAI image tools.
10. Which one should I choose in 2025?
A.: Choose ChatGPT for creativity and tools. Choose Bing AI for search and speed.
Conclusion and Summary – ChatGPT vs Bing AI
ChatGPT and Bing AI are impressive tools powered by the same GPT engine, but their similarities end here.
ChatGPT excels in creative writing, flexible use cases, and community trust. Its rapid evolution—especially with GPT-4o—offers unmatched speed, advanced tools (like a code interpreter), and plugin support.
It behaves like a co-pilot for creators, researchers, developers, and anyone needing deep, nuanced conversations.
Bing AI, by contrast, is tailored for web integration. If you focus on live search, quick answers, or visual browsing, Bing AI’s web-native nature gives it an edge.
It’s ideal for general users, especially those inside the Microsoft ecosystem. Still, the tool feels more like an advanced FAQ bot than a true conversational partner.
Regarding social presence, Bing AI is embedded directly in public-facing systems like Bing Search and Microsoft Edge, making it far more visible to the average user.
While widely used, ChatGPT operates behind a login wall—it is more private but less “publicly discoverable.”
Choose ChatGPT if you value fluid conversation, creative generation, and productivity tools.
Go with Bing AI if your needs are closer to search, citations, or fast answers from the web.
This article is part of the AI Tools Comparison Series (Revolutionizing AI: Top Tools and Trends; it can be found here: Emerging Technologies).
Related Posts – ChatGPT vs Bing AI
Based on your current Digital Chronicle topics:
- ChatGPT vs 11 Powerful AI Tools – What’s the Difference? ⬈
- Discover the Evolution of Artificial Intelligence from the 19th Century ⬈
- Cybersecurity in AI-Based Workflows: Unstoppable Deep Dive in 2024? ⬈
- Augmented Reality vs Virtual Reality: Revolutionary Tech in 2024 ⬈
Thanks for reading.
Resources – ChatGPT vs Bing AI
ℹ️ note: Due to the ongoing development of applications and websites, the actual appearance of the websites shown may differ from the images displayed here.
The cover image was created using Leonardo AI.