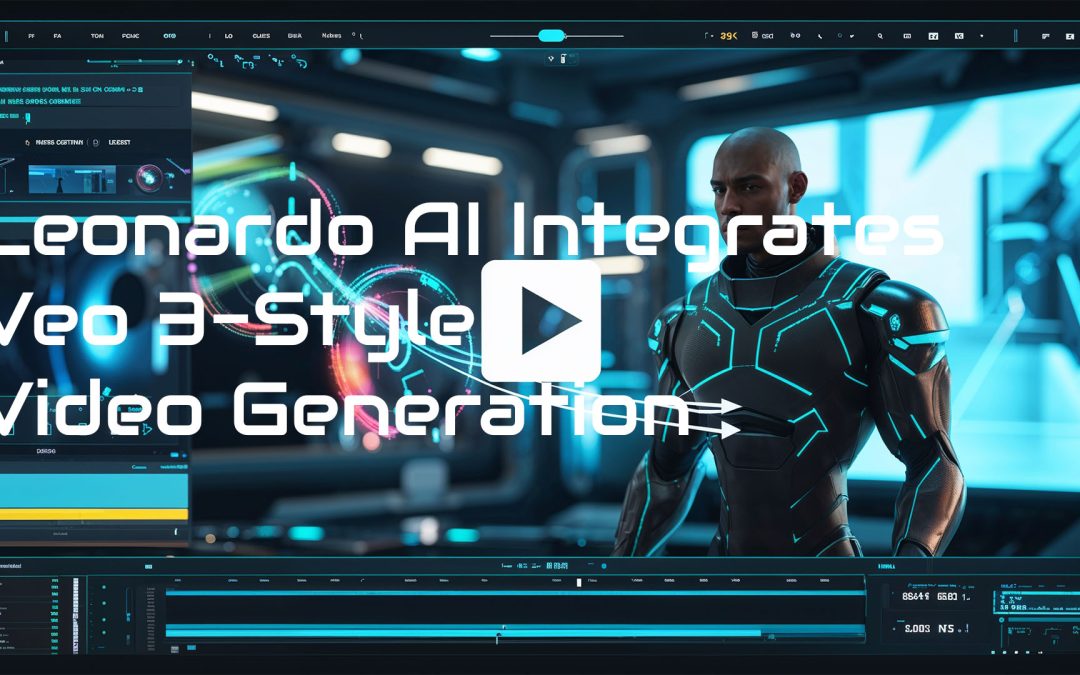
Leonardo AI Integrates Veo 3: 5 Stunning Features Unveiled
Introduction – Leonardo AI Integrates Veo 3
Artificial Intelligence in video creation just crossed a new frontier.
Leonardo AI, once known for its top-tier image generation, has now introduced Veo 3-style capabilities, enabling creators to transform still images into cinematic video scenes with motion control, camera dynamics, and character animation.
This breakthrough puts Leonardo in direct competition with tools like OpenAI’s Sora, Runway ML, and Pika Labs.
For content creators, educators, marketers, and indie developers, this update is not just incremental—it’s revolutionary. It bridges the gap between static image generation and full video storytelling within a user-friendly workflow.
Let’s explore how Leonardo’s new video engine works, what it can do, and how it compares to today’s most powerful AI video generators.
What is the Veo 3-Style Video Feature in Leonardo AI?
The new feature, currently accessible under Leonardo Video or Motion Control, lets users generate short, animated clips from static images or text prompts.
This mirrors what tools like Sora and Runway do—but directly within Leonardo’s sleek interface.
5 Stunning Features – Leonardo AI Integrates Veo 3:
- Dynamic camera movement (zoom, pan, rotate)
- Start-frame control (select your image, use it as a scene base)
- Motion path overlay (choose how and where the motion unfolds)
- Emotion-aware facial expressions (in development)
- 16:9, 9:16, and 1:1 aspect ratio support
It’s like turning a MidJourney image into a short cinematic video, with total creative control.
How It Works: From Prompt to Motion
Creating video content in Leonardo follows this general flow:
- Start with an image or text prompt
Use a pre-generated image or create one from scratch using Leonardo’s standard tools. - Switch to the “Video” tab.
Choose the new video workspace with Motion Path, Camera Controls, and Frame Management tools. - Add dynamic elements
Set camera behavior (tracking shot, push-in, or pan). Soon, you can animate subjects via facial or body gestures. - Render and export
Output a short MP4 clip (4–8 seconds) ready for YouTube Shorts, TikTok, Reels, or editing in Filmora/DaVinci Resolve.
This simplicity allows for massive scaling in content production, especially for creators on a tight schedule.
Comparison Table: Leonardo vs Sora vs Runway vs Pika
Feature / Tool | Leonardo AI | Sora (OpenAI) | Runway ML | Pika Labs |
---|---|---|---|---|
Text-to-Video | ✅ | ✅ | ✅ | ✅ |
Image-to-Video | ✅ | ✅ | ✅ | ✅ |
Motion Path Control | ✅ | ❌ (hidden) | ⚠️ Basic | ✅ |
Dynamic Camera Movement | ✅ | ✅ | ✅ | ✅ |
Talking Face / Voice Sync | ⚠️ In dev. | ✅ | ✅ | ⚠️ Partial |
Resolution Options | 720p–1080p | Up to 1080p | Up to 4K | Up to 1080p |
Export Format | MP4 | MP4 | MP4, MOV | MP4 |
Free Tier | ✅ | ❌ | ✅ | ✅ |
Commercial Use Allowed | ✅ | ✅ | ✅ | ✅ |
Ideal For | Creators | Studios | Creators | Creators |
Who Should Use Leonardo’s Video Engine?
This Tool is Ideal for:
- YouTubers & TikTokers creating short-form video content
- Educators illustrating concepts with animated visuals
- Startups needing fast, high-quality promo videos
- Indie developers prototyping cinematic game scenes
- AI creatives building multi-modal storytelling projects
Leonardo offers low entry barriers, quick rendering, and a simple interface—perfect for beginners and pros alike. The fact that Leonardo AI Integrates Veo 3-style video creation is a significant advancement in technology.
❓ FAQs – Leonardo AI Integrates Veo 3
What is Leonardo AI’s new video feature?
Leonardo AI now supports motion video generation, enabling users to animate static images with motion paths and camera movements.
Is this similar to OpenAI’s Sora?
Yes, Leonardo AI now offers comparable capabilities such as dynamic motion, though Sora still leads in realism and voice synchronization.
Can I use my own images?
Yes, you can upload your own image or generate one within Leonardo to serve as the base for your animation.
What output formats are supported?
Leonardo currently exports videos as MP4 in standard resolutions suitable for Shorts, TikTok, and YouTube.
Does it support text-to-video prompts?
Yes, Leonardo supports both text-based generation and image-to-video workflows.
How long are the generated videos?
Typical outputs are 4–8 seconds long, but batch rendering and looping are possible for longer projects.
Are there facial animations or talking videos?
Facial animation is developing early; talking video support is planned but not yet public.
Can I use this commercially?
Yes, content created with Leonardo AI is available for commercial use based on their terms.
How much does it cost?
Leonardo offers free and paid tiers. The video feature is accessible even with free credits, though usage may be limited.
Can I export and edit the videos elsewhere?
Yes, the output MP4s can be imported into editors like DaVinci Resolve, Filmora, or Adobe Premiere for further enhancement.
🧠 Conclusion & Summary – Leonardo AI Integrates Veo 3
Leonardo’s integration of Veo 3-style video generation marks a turning point. It gives everyday creators access to cinematic AI storytelling tools that were once only possible with powerful studio-grade models.
With camera control, motion path overlays, and seamless rendering, Leonardo is among the top players in AI-generated video.
Whether you’re producing YouTube Shorts, enhancing product presentations, or testing AI storytelling, this feature effortlessly brings your visuals to life.
The AI video revolution just got real. And it’s in your hands now.
Related Posts
- Create Sora-Style AI Videos for Free on Android & iOS in 2025 ⬈
- InVideo IO vs Video Express AI: A Comprehensive Comparison in 2024 ⬈
- Adobe Firefly vs Leonardo AI: Unleashing Creative Brilliance in 2024 ⬈
- Discover 15 Video Editors: A Comprehensive Comparison in 2024 ⬈
This article is part of the AI Tools Comparison Series (Revolutionizing AI: Top Tools and Trends, it can be found here: Emerging Technologies).
Thanks for reading.
📚 Resources & Further Reading – Leonardo Integrates VEO 3
Explore the tools behind the AI video revolution. Here are the official pages and partner platforms to get started or dive deeper.
- Access Leonardo AI with Official Partner Benefits (via Digital Chronicle)
- OpenAI Sora – Product Overview ⬈
- Runway ML – Multimodal Video Creation ⬈
- Pika Labs – AI-Powered Video Tool ⬈
- ElevenLabs – Voice Cloning and Narration Tools ⬈
ℹ️ Note: Due to the ongoing development of applications and websites, the actual appearance of the websites shown may differ from the images displayed here.
The cover image was created using Leonardo AI.