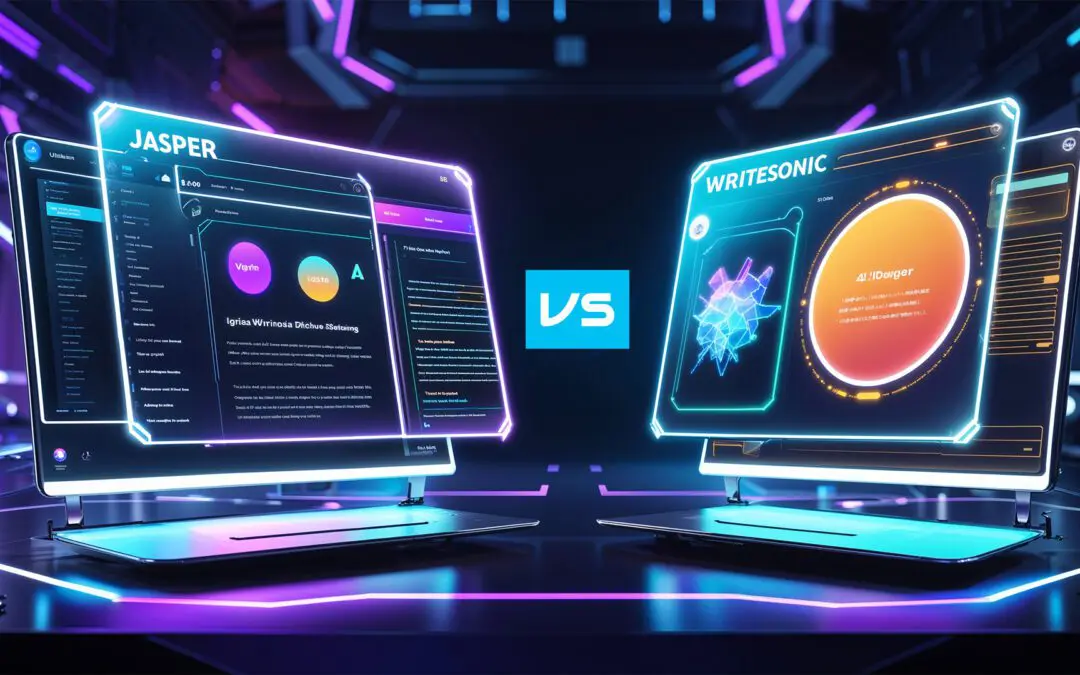
Jasper vs Writesonic: Discover Which AI Writing Tool Reigns Supreme in 2025?
Introduction – Jasper vs Writesonic
AI writing tools have revolutionized content creation, offering speed, efficiency, and SEO-friendly text generation.
Two major contenders, Jasper and Writesonic, stand out in this competitive space. But which one is better for your needs?
This detailed comparison covers their features, pricing, SEO capabilities, community engagement, and more.
Features Comparison – Jasper vs Writesonic
1. User Interface and Ease of Use
Jasper – The AI Copywriting Specialist
Jasper offers an intuitive dashboard with a clean, user-friendly interface. It’s designed for content marketers, bloggers, and businesses seeking high-quality, long-form content.
Writesonic – AI Article Writer & AI Marketing Agent
2. AI Models and Content Quality
- Jasper: Uses GPT-4, ensuring high-quality, coherent, and in-depth content generation.
- Writesonic: Offers both GPT-3.5 and GPT-4, giving users flexibility depending on their budget and content requirements.
3. SEO Optimization
Both tools excel in SEO content generation:
- Jasper integrates with Surfer SEO (learn more about Surfer SEO in the Resouces Section), making it a powerhouse for optimized articles.
- Writesonic provides built-in keyword suggestions and meta-tag optimization.
4. Templates and Customization
- Jasper has over 50+ templates, including blog posts, ad copy, and email sequences.
- Writesonic provides over 80+ AI templates, emphasizing ad copy, landing pages, and social media content.
5. Plagiarism and Grammar Check
Both tools come with built-in grammar checks. However, Jasper integrates with Grammarly, offering an extra layer of proofreading.
Pricing Plans – Jasper vs Writesonic
Jasper
- Starter Plan: $39/month (Basic AI copy)
- Boss Mode: $59/month (Advanced AI writing + SEO tools)
- Business Plan: Custom pricing for enterprises
Writesonic
- Free Plan: Limited usage with GPT-3.5
- Pro Plan: Starts at $20/month (GPT-4 access)
- Enterprise Plan: Custom pricing with API access
SEO Role of Jasper and Writesonic
Jasper’s SEO Strengths
- Integrates with Surfer SEO for real-time keyword optimization.
- Generates in-depth, authoritative content for blog posts and articles.
- Helps in creating structured content with proper headings and subheadings.
Writesonic’s SEO Strengths
- Built-in SEO tools for keyword research and on-page optimization.
- Generates SEO-friendly meta descriptions and titles.
- Fast content generation for blog outlines and social media posts.
Community Engagement and Support – Jasper vs Writesonic
- Jasper has an active Facebook community, a strong knowledge base, and responsive customer support.
- Writesonic engages users via its Facebook group, Discord server, and live webinars.
FAQs – Jasper vs Writesonic
- Which tool is better for long-form content?
A.: Jasper excels in generating high-quality, in-depth articles. - Which AI is better for ad copy and social media?
A.: Writesonic is designed for short-form, marketing-focused content. - Do Jasper and Writesonic offer free trials?
A.: Writesonic has a free plan, while Jasper offers a limited trial. - Which tool is better for SEO content?
A.: Jasper, with Surfer SEO integration, is superior for long-form SEO content. - Does Writesonic support multiple languages?
A.: Yes, Writesonic supports over 25 languages. - Can I use Jasper for email marketing?
A.: Yes, Jasper offers AI-generated email sequences and templates. - Is Writesonic cheaper than Jasper?
A.: Writesonic has more budget-friendly options starting at $20/month. - Which AI provides more templates?
A.: Writesonic offers 80+ templates, whereas Jasper has around 50. - Do these tools have plagiarism checkers?
A.: Both have plagiarism detection, but Jasper integrates with Copyscape. - Which tool is best for small businesses?
A.: Writesonic’s affordable plans make it ideal for small businesses.
Conclusion and Summary – Jasper vs Writesonic
Both Jasper and Writesonic are powerful AI writing tools catering to different audiences.
If you need high-quality, long-form content with strong SEO capabilities, Jasper is the better choice, especially with its Surfer SEO integration and superior content structure.
On the other hand, if you require fast, engaging, short-form content for ads, social media, and product descriptions, Writesonic is a more budget-friendly and efficient solution.
Pricing also plays a crucial role. Writesonic is more affordable, making it accessible to startups and freelancers. Jasper, while expensive, delivers premium-quality content, making it ideal for businesses and marketers who need top-tier SEO articles.
Both tools offer community support, frequent updates, and powerful AI-driven writing capabilities. Ultimately, your choice depends on your specific content needs.
If you prioritize in-depth, SEO-optimized blogs, choose Jasper. If you need cost-effective, quick AI-generated copy, use Writesonic.
This article is part of the AI Tools Comparison Series (Revolutionizing AI: Top Tools and Trends; it can be found here: Definitive Guide to Brilliant Emerging Technologies in the 21st Century).
For a brief comparison on the subject, see my previous post, ChatGPT vs. 11 Powerful AI Tools: Unlock Their Unique Features in 2024
Thanks for reading.
Resources
- Jasper – The AI Copywriting Specialist ⬈
- Writesonic – AI Article Writer & AI Marketing Agent ⬈
- Surfer – SEO Content Optimization Platform ⬈
Surfer is an all-in-one platform for keyword research, content creation, and optimization. It has been synonymous with SEO since 2017, and with the launch of Surfer AI, we’ve made scaling and ranking content more effortless than ever.
ℹ️ note: Due to the ongoing development of applications and websites, the actual appearance of the websites shown may differ from the images displayed here.
The cover image was created using Leonardo AI.