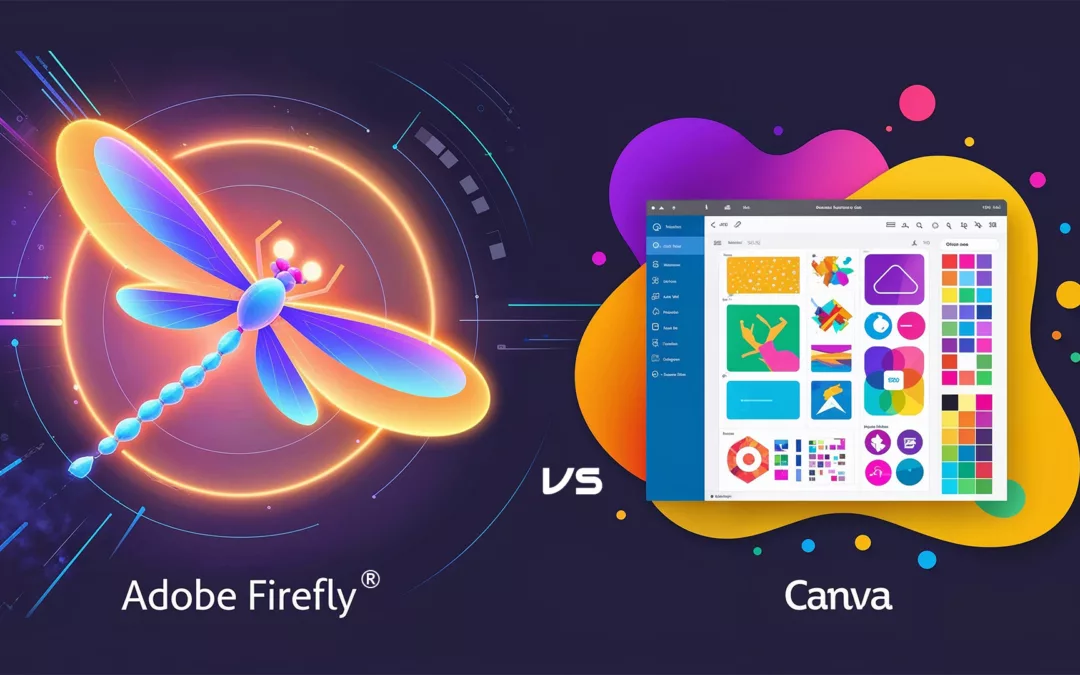
Adobe Firefly vs Canva: A Comprehensive Creative Duel in 2025
Introduction – Adobe Firefly vs Canva
In the rapidly evolving world of design tools, two platforms have emerged as frontrunners in redefining digital creativity: Adobe Firefly and Canva. Adobe Firefly, a relatively new player, harnesses the power of generative AI to streamline creative workflows. Canva, on the other hand, is a versatile platform cherished for its user-friendly approach and affordability.
This article explores both tools’ strengths, weaknesses, and prices, helping you choose the best suits your needs.
What is Adobe Firefly?
Adobe Firefly® is a generative AI tool integrated into the Adobe Creative Cloud ecosystem. It offers powerful features like text-to-image generation, content-aware fills, and AI-assisted design workflows.
Key Features
- Text-to-Image Generation: Turn simple text prompts into stunning visuals.
- Content-Aware Fill: Intelligently fill gaps or remove objects from images.
- Style Transfer: Apply artistic styles to your content seamlessly.
- Adobe Ecosystem Integration: Works flawlessly with Photoshop, Illustrator, and Premiere Pro.
Pricing
Adobe Firefly is included in Creative Cloud subscriptions:
- Individual Plan: $54.99/month (includes access to all Creative Cloud apps).
- Business Plan: $84.99/month per user.
- Free Trial: 7-day trial available.
What is Canva?
Canva is a design platform renowned for its simplicity and accessibility. It suits both professionals and beginners and makes graphic design easier and more collaborative.
Key Features
- Template Library: Thousands of ready-to-use templates for social media, presentations, and more.
- Drag-and-Drop Editor: Intuitive tools for creating designs quickly.
- Collaboration: Real-time collaboration for teams.
- Free and Paid Plans: Offers robust features even in the free tier.
Pricing
Canva offers three pricing tiers:
- Free Plan: Includes basic features and templates.
- Pro Plan: $12.99/month or $119.99/year (billed annually).
- Enterprise Plan: Custom pricing for large organizations.
Adobe Firefly vs Canva: Feature-by-Feature Comparison
1. Ease of Use
- Adobe Firefly: Designed for professional users with advanced features but a steeper learning curve.
- Canva: Ideal for beginners and non-designers due to its intuitive interface.
2. AI Capabilities
- Adobe Firefly: Excels in generative AI, offering unmatched precision and innovation.
- Canva: Limited AI features primarily focused on basic automation.
3. Collaboration
- Adobe Firefly: Supports collaboration but requires Creative Cloud licenses for all team members.
- Canva: Superior in team collaboration with features available even in the free plan.
4. Cost-Effectiveness
- Adobe Firefly: Best suited for professionals and organizations willing to invest.
- Canva: Offers affordable plans, making it a favorite for startups and educators.
Pros and Cons – Adobe Firefly vs Canva
Adobe Firefly
Pros:
- Advanced AI tools.
- Seamless integration with Adobe’s ecosystem.
- High-quality output.
Cons:
- Expensive.
- Requires design expertise.
Canva
Pros:
- Budget-friendly.
- Easy to use for non-designers.
- Rich template library.
Cons:
- Limited AI capabilities.
- Not suitable for advanced design projects.
Pricing Comparison Table – Adobe Firefly vs Canva
Feature | Adobe Firefly | Canva |
---|---|---|
Starting Price | $54.99/month | Free |
AI Features | Advanced | Basic |
Collaboration | Requires licenses | Free and Pro Plans |
Best For | Professionals | Beginners/Teams |
Connection with Discord
Adobe Firefly and Canva have established integrations with Discord to enhance collaboration and community engagement.
Adobe Firefly leverages Discord to connect with its community of designers, offering channels where users can share creations, discuss AI-powered workflows, and receive support directly from Adobe experts.
Canva also maintains a strong presence on Discord, where educators, marketers, and content creators can share templates, exchange design tips, and collaborate in real time. These integrations make it easier for users to engage with peers and industry professionals, fostering a dynamic creative ecosystem.
FAQs – Adobe Firefly vs Canva
- What is Adobe Firefly’s primary advantage over Canva?
A.: Adobe Firefly excels in advanced AI-driven design capabilities. - Can I use Canva for free?
A.: Yes, Canva offers a free plan with limited features. - Is Adobe Firefly available as a standalone app?
A.: No, it’s part of Adobe Creative Cloud. - Which tool is better for team collaboration?
A.: Canva offers better collaboration features, especially for small teams. - Does Adobe Firefly support mobile platforms?
A.: Yes, it integrates with Adobe’s mobile apps. - Can Canva create videos?
A.: Yes, Canva supports basic video creation. - Which platform is more cost-effective for educators?
A.: Canva, with its free and affordable Pro plans, is more cost-effective. - Does Adobe Firefly have a free plan?
A.: No, only a 7-day free trial is available. - Can I use Canva without any design experience?
A.: Yes, it’s designed for beginners. - Which platform offers better templates?
A.: Canva has a larger library of ready-to-use templates.
Conclusion and Summary – Adobe Firefly vs Canva
Adobe Firefly and Canva cater to different audiences. Adobe Firefly is a powerhouse for professionals who require advanced tools and seamless integration within Adobe’s ecosystem. However, it comes at a premium cost. Canva is affordable and easy to use, making it ideal for beginners and small teams.
Adobe Firefly is your go-to if you’re a professional designer looking for cutting-edge AI features. For those seeking a budget-friendly, user-friendly tool for more straightforward projects, Canva is unbeatable.
This article is part of the AI Tools Comparison Series (Revolutionizing AI: Top Tools and Trends; it can be found here: Emerging Technologies).
Thanks for reading.
Resources – Adobe Firefly vs Canva
Canva ⬈
Visit Canva’s homepage to explore subscription plans, showcases, and detailed tutorials for generating hyper-realistic AI art.
Discord ⬈
Explore the hub of creativity for Leonardo AI users and engage with an active community of artists, sharing tips, creations, and discussions.
ℹ️ note: Due to the ongoing development of applications and websites, the actual appearance of the websites shown may differ from the images displayed here.
The cover image was created using Leonardo AI.